Enabling Technologies in Agriculture: Outsized Returns Across the Value Chain?
There are constant murmurs about agriculture being on the cusp of another green revolution but this time it’s different. Enabling technologies are converging to deliver results greater than the sum of their parts and farming is seeing significant change, including:
- Artificial intelligence is driving more effective and timely decisions
- Computational biology is changing what we put in soils to improve yield
- Automation is trying mechanization to keep pace with the twin headwinds of a decreasing farm labor population and the increasing size and of farms.
- Aerial imagery-whether from drones, satellites or the hyperspectral-is providing a new data stream to support these decisions.
- In synthetic biology, Next Generation Sequencing (NGS) or editing technologies like CRISPR are increasing the pace and accuracy of biological alternatives to agrochemicals.
What is clear is that agriculture is one of the last industries to digitize, and thus, one of the last to reap the benefits of many of these technologies. However, as we see venture investment targeting agtech in record volumes (Q3 2020 saw over $2.5 billion invested), perhaps we will see agriculture start to move up this curve.
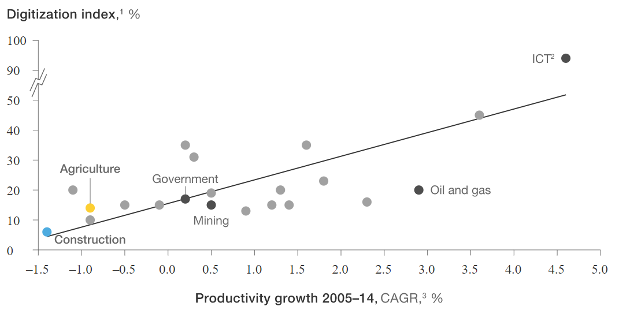
So, which enabling technologies are we looking at, exactly? Below is an overview of some of the technologies we have focused on in our Ag & Food market research service.
Artificial Intelligence
The attractiveness of investing in AI solutions for agriculture knows no bounds. The widespread use of Artificial Intelligence (AI) in broad acre arable farming will be the most significant transformation to the arable industry since the adoption of the tractor. According to Cisco’s visual networking index, 11.6 billion devices are connected to the Internet. The data insights from these devices carry an $11 trillion economic value when combined with some method of interpretation, i.e. artificial intelligence.
In agriculture, the variety of business models being built on AI engines is unsurprisingly vast. From the original agtech. unicorn The Climate Corporation using AI to drive insights from agronomic data and improve precision agriculture, to companies like AI Palette trying to predict consumer food trends to optimize manufacturing and product prototyping, there is a wealth of data to be mined.
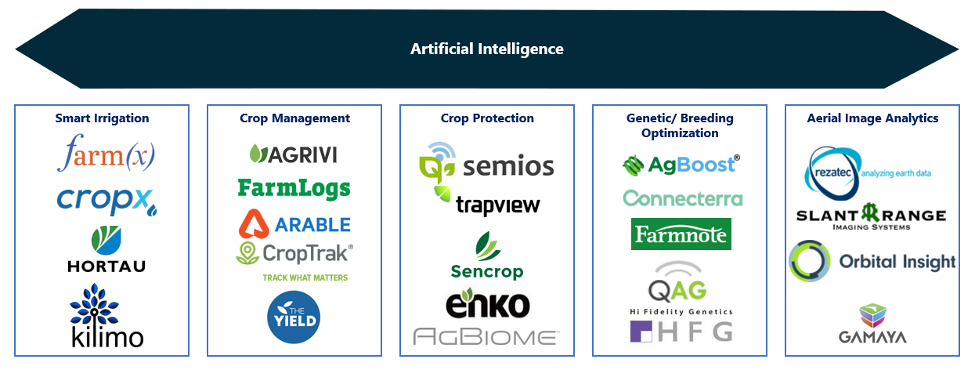
As we can see, the AI playbook is being used in a broad range of cases, often using entirely new data streams in a similar way that satellite data analytics companies do. For a more niche application, we interviewed AgBoost. The company is taking all available data on the size and quality of cattle and combining it with genomic data to optimize breeding decisions. Typically, the industry’s approach had been visual inspection, with results taking two to three years of inspecting the animal’s growth to discern if you were right. At this point, the farmer would have spent up to $3,000 on that cow’s growth, with a 50% industry standard chance of being wrong about whether the cow had the desired genetic traits. AgBoost is using an AI engine to analyze data and present it to breeders through a subscription service at ~$6/animal/year.
Automation with Advanced Robotics
While automation is really a subset of AI, when it combines with robotic hardware it creates a new category of enabling technology. The benefits of automation of agricultural tasks include:
- Quality and accuracy of process
- Reduced cycle times and increased productivity
- Improved compliance in industrial systems
- Improved cleanliness and sanitation
- Reduced crop maintenance and harvesting cycle times
- Operating cost savings.
In agriculture, there are some strong amplifiers of these benefits, including the global farm labor crisis due to low wages, difficult conditions and the trend of workforces migrating to cities. Plus, environmental concerns put pressure on farm systems to apply irrigation, fertilizers, pesticides and other farm inputs more precisely. Finally, there are concerns about food safety and supply chain resiliency.
There are four main revenue models for robotics developers:
- Direct sales of robots to farmers
- A Robotics-as-a-Service (RaaS) model to deliver and operate robots on site as required
- A Software-as-a-Service (SaaS) model for online software upgrades for crop analytics and insights derived from the robot’s sensors and machine vision data
- Aftermarket sales of parts and services.
The revenue per robot is likely to be higher with the as-a-service model. This is because you can add various layers of service onto one robotic platform, including crop mapping, weeding or anti-fungal UV light treatment. This also reduces economic risk to the farmer, who may pay the same per hectare price for a robotic service as per hectare chemical treatment. While this is the favored revenue model, subsidies and availability anxiety are driving direct sales of robots.
Developers of robotics compete between single and multi-functional robots, specific crop types and operating location. While Ecorobotix are focusing on single function (weeding), Saga Robotics have built an autonomous platform to which multiple tools can be attached to perform different tasks. This differs from a company such as ZTractor, which is trying to replace existing tractors with autonomous electric vehicles, or Agrointelli, which is trying to bolt existing equipment onto a new robotic platform to accelerate adoption.
It is worth noting that incumbent farm machinery providers are on the verge of losing their competitive advantage over start-ups. While they generally remain the most trusted source of new technologies, key technologies such as autonomous steering and connectivity to farm management software are often seen as being handled more capably by startups.
Computational Biology
Synthetic biological crop inputs have been available for decades. However, adoption has been hampered due to issues with efficacy, shelf life and long, expensive R&D cycles. New technology is enabling more effective microbial crop inputs. Faster trait discovery can happen by using machine learning and automated laboratory systems coupled with quicker and cheaper genomic sequencing technology. This accelerates the discovery of new microbes and the isolation of new genetic traits. Companies such as AgBiome are building machine learning engines accelerating the discovery of beneficial microbes. Similarly, Inari is using trait discovery engines to accelerate the discovery of desirable genetic traits and the development of new seed variety.
While the capabilities of these discovery engines continue to grow, improvements in technology have increased the speed at which these tests can be carried out. This has provided the capacity for on-site genetic testing. Clear Labs was leveraging Nest Generation sequencing technology to provide on-site food testing before it pivoted to try and deploy its technology as a Covid-19 diagnostic test. Similar technology is being developed in the field of soil science with companies like Trace Genomics. This increase in testing speed and accuracy will accelerate precision agriculture techniques and set the scene to become a key building block in the ecosystem of service payments for agricultural soil stewardship.
Big Tech in AgTech
Technology and telecom giants are building platforms for AI, robotics and agricultural analytics engines. For startups, this translates to either strong competition against established high-tech brands or an increased number of routes to exit. For example, the Canadian telecoms giant Telus made a strategic decision to build an agtech platform through acquisition. This led to the company acquiring AFS Technologies, AGIntegrated, Agrian, Decisive Farming, Farm at Hand, Muddy Boots, and TKXS within the last 18 months. This makes sense for a telecom giant looking to capitalize on the last industry to connect to the internet and unlock the potential of enabling technologies mentioned here. Similar acquisition strategies can be seen with Trimble (Digi, MagGrow, Cityworks) or Raven (Smart Ag, DOT Automation, Agsync).
Large tech companies are also looking at the agriculture industry and seeing opportunities to grow. Watson Decision Platform for Agriculture was released by IBM Watson and The Weather Company, combining agriculture with IBM’s advanced capabilities in Artificial Intelligence, Internet of Things and Cloud Computing. Microsoft have been working on its FarmBeats platform for five years, offering it as part of its cloud services platform Azure. Google X also revealed its Mineral Project, building on know-how from Waymo to build an agricultural robot.
Challenges
A number of key challenges remain but there are two major ones. The first is connectivity on the farm. If connectivity is implemented successfully, the industry could add $500 billion in additional value to the global gross domestic product by 2030. The second key challenge is a regulatory framework the encourages the development and adoption of these technologies. When speaking to agricultural robotics developers, there is some frustration with existing subsidies granted for buying large pieces of machinery. While this improves their short-term sales, the long-term subscription model is hampered. It also reduces the potential for a shared economy approach to machinery. Incentivizing ecosystem service payments (as proposed in the UK) could advance the adoption of highly granular precision agriculture tools, tools that employ robots to feed AI engines that will drive not only soil health and crop productivity but long-term agricultural prosperity as well.